
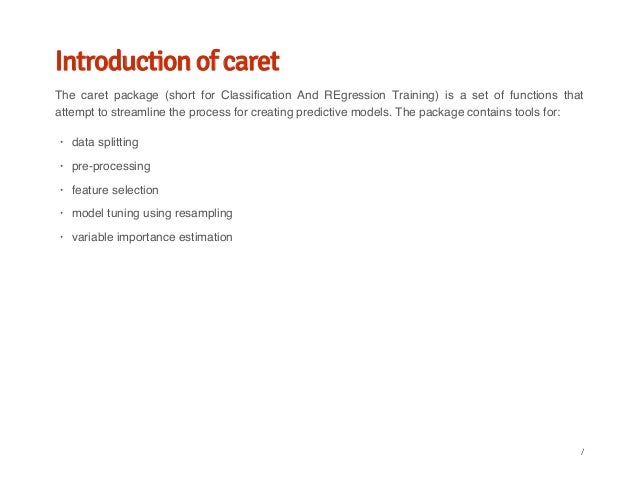
Split data (Train/Test, Cross-Validation)īoth packages provide functions for common data splitting strategies, such as k-fold, grouped k-fold, leave-out-one, and bootstrapping. bike_all$season <- factor( bike_all$season, levels = c(1, 2, 3, 4), labels = c("spring", "summer", "autumn", "winter") ) bike_all$holiday <- factor( bike_all$holiday, levels = c(0, 1), labels = c(FALSE, TRUE) ) bike_all$workingday <- factor( bike_all$workingday, levels = c(0, 1), labels = c(FALSE, TRUE) ) bike_all$weather <- factor( bike_all$weather, levels = c(1, 2, 3, 4), labels = c("clear", "cloudy", "rainy", "heavy rain"), ordered = TRUE ) head(bike_all) bike_all % select(-casual, -registered) # Original skewness(bike_all$total) # 1.277301 # Log skewness(log10(bike_all$total)) # -0.936101 # Log + constant skewness(log1p(bike_all$total)) # -0.8181098 # Square root skewness(sqrt(bike_all$total)) # 0.2864499 # Cubic root skewness(bike_all$total^(1 / 3)) # -0.0831688 # Transform with cubic root bike_all$total <- bike_all$total^(1 / 3) PredictorsĬategorical variables are converted to factors according to the attribute information provided by UCI. I tried several common techniques for positively skewed data and applied the one with the lowest skewness - cubic root.
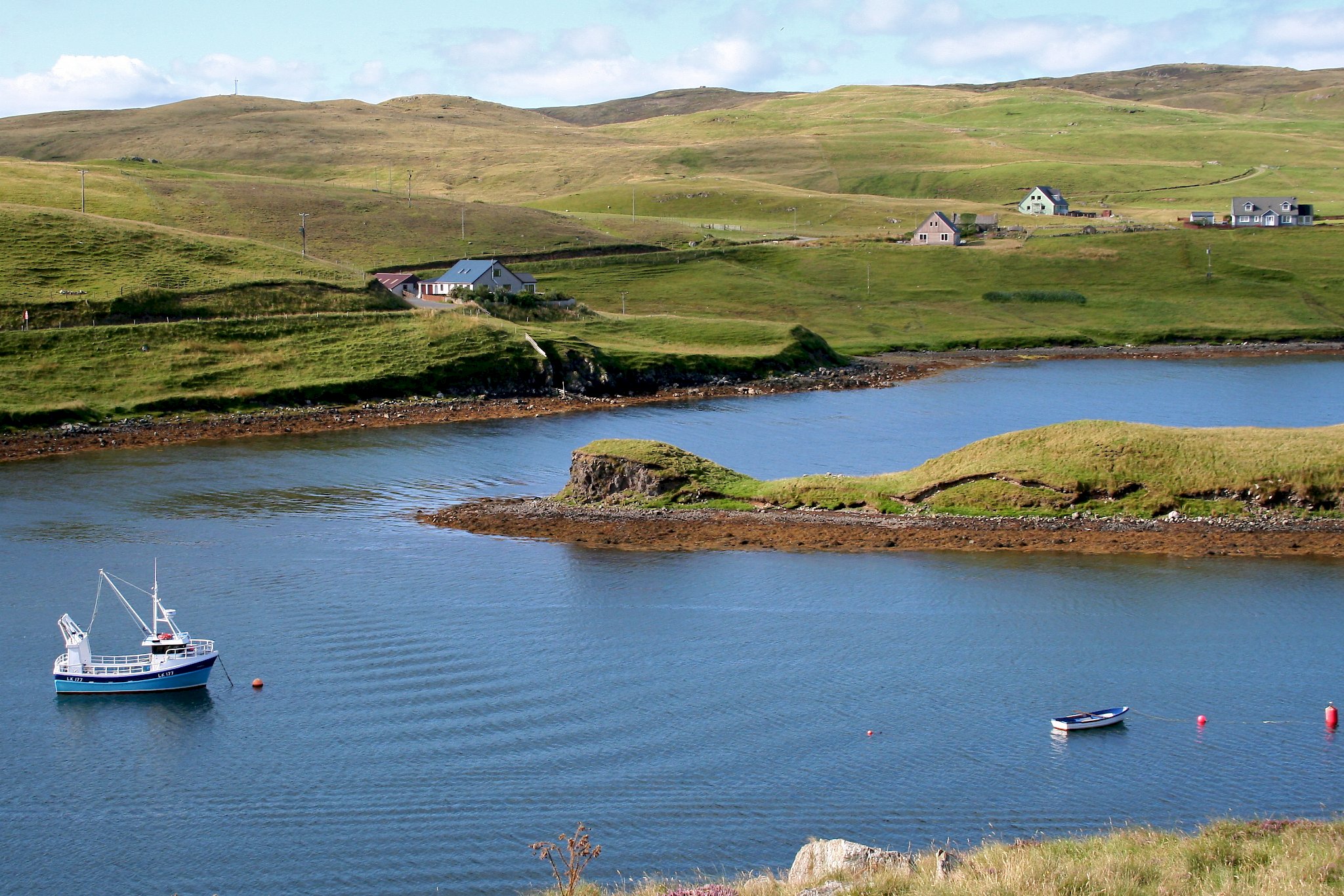
As suggested earlier, the target variable is positively skewed and requires transformation. I focused on the total count, so casual and registered variables are moved. However, for normalisation, I need to know the minimum and the maximum value of a variable, both of which might be different for training and testing. For example, if I take the square root of a number, I can square it to know the original number. Here, I focus on the process that applies to all data and does not have a parameter, such as factorising or simple mathematic calculation. Since I have not split the data yet, this step is not data scaling or centring, which should fit the training set and transform the testing set. However, for a beginner, it might be intimidating (at least it was for me). This is beneficial for users because of the increased flexibility and possibility. As shown, tidymodels breaks down the machine learning workflow into multiple stages and provides specialised packages for each stage. Some common libraries from tidyverse, such as dplyr, are also loaded. dials: for creating and managing tuning parameters.broom: for converting the information in common statistical R objects into user-friendly, predictable formats.workflow: for putting everything together.parsnip: for trying out a range of models.rsample: for data splitting and resampling.When I execute the library(tidymodels) command, the following packages are loaded: Tidymodels is a collection of packages for modelling. For example, createDataPartition for splitting data and trainControl for setting up cross-validation.

in Ecology from Dartmouth College and lives in Boston with his wife, Lindsay, and dog, Moose.Caret is a single package with various functions for machine learning. Zach is a co-author of the () for machine learning and the author of the () for ensemble learning. He is a highly successfully Kaggler who has been working at data science startups and consulting firms for 7 years. Zach Deane-Mayer is the Lead Data Scientist at Cognius. Zach Deane-Mayer – Lead Data Scientist at Cognius He and Kjell Johnson published the bestselling book () in 2013. Max’s interests are in predictive modeling and machine learning and is the author of six R packages, including the (). He has worked in pharmaceutical and molecular diagnostic research for more than 15 years. Max Kuhn is a Director of Nonclinical Statistics in Pfizer R&D. Max Kuhn – Director of Statistics at Pfizer R&D Friction points with CRAN and their resolution will also be discussed. In this talk, we will outline the somewhat unique aspects of the package and how it impacts the development environment (including documentation and testing).
Caret package code#
The caret package is a unified interface to a large number of predictive model functions in R.įirst created in 2005, the home for the source code and documentation has changed several times. The caret package is a unified interface to a large number of predictive model functions in R.
